The fields of biology and data science have a lot in common. Data scientists and biologists both analyze datasets to try to make sense of the world. Today, data science is becoming increasingly important for biology, as biologists increasingly use machine learning and AI for drug discovery, medical diagnosis, and automating repetitive tasks.
Nonetheless, there is still a large cultural divide between the two fields. Data scientists and biologists regularly approach the same problem from very different perspectives, using different methods and different terminology.
We chatted to Alexander Titus, Chief Strategy Officer (CSO) at the Advanced Regenerative Manufacturing Institute (ARMI) and editor in chief of Bioeconomy.XYZ. Alexander started out as a biologist, then obtained a PhD in data science, and now works towards bridging the gap between the two fields.
Alexander’s background
Alexander first studied biochemistry and biology in college, but was introduced to computer science in his last semester. As he puts it,
“I fell in love with computer science and realized I had studied the wrong thing the whole time.”
“I moved to Silicon Valley and wanted to get a job at a startup in the tech world. But I didn't have any software skills, so I was working on things unrelated to programming. Then in true Silicon Valley style, I spent all of my time hacking away in my basement: Learning how to code. Learning statistics. Eventually learning machine learning. I got good enough that I could go get my PhD.”
Now, as a strategy executive, Alexander acts as a bridge between data scientists and biologists. He helps lead ARMI towards the right big-picture decisions, making regenerative manufacturing a reality.
[Find out more about Alexander on LinkedIn, Twitter, his podcast Titus Talks, his publication Bioeconomy.xyz, or at alexandertitus.com.]
Should biologists learn data science, or should data scientists learn biology?
Alexander has seen people broadening their skillset in both directions, but he thinks it’s easier for biologists to gain data science skills than vice-versa. He says:
“The founders I talk to find it easier to take biologists and teach them math and programming than to take people with a long history in math and science – but not in biology – and try to catch them up on years of facts and information they've missed out on.”
But either direction is challenging, and Alexander says a solid grounding in data science fundamentals is useful for anyone who wants to keep pace with the constant changes:
“Programming changes so fast. Python was huge, and then R was integrated. Then all the statistics, then TensorFlow, then Keras. It just changes constantly. Any one set of skills are obsolete in a matter of months. But the underlying ability to learn those skills is huge.”
In fact, Alexander believes “learning to learn” is the most important thing college teaches people:
“I had a professor in college who always used to tell me, ‘The only thing college teaches you is how to learn anything in a long weekend.’ That's the mindset I really need from people. It doesn't matter what it is, because someone's going to invent some new back propagation algorithm, or some new framework we're going to have to use. We're always going to have to adapt.”
“A cultural difference in explainability”
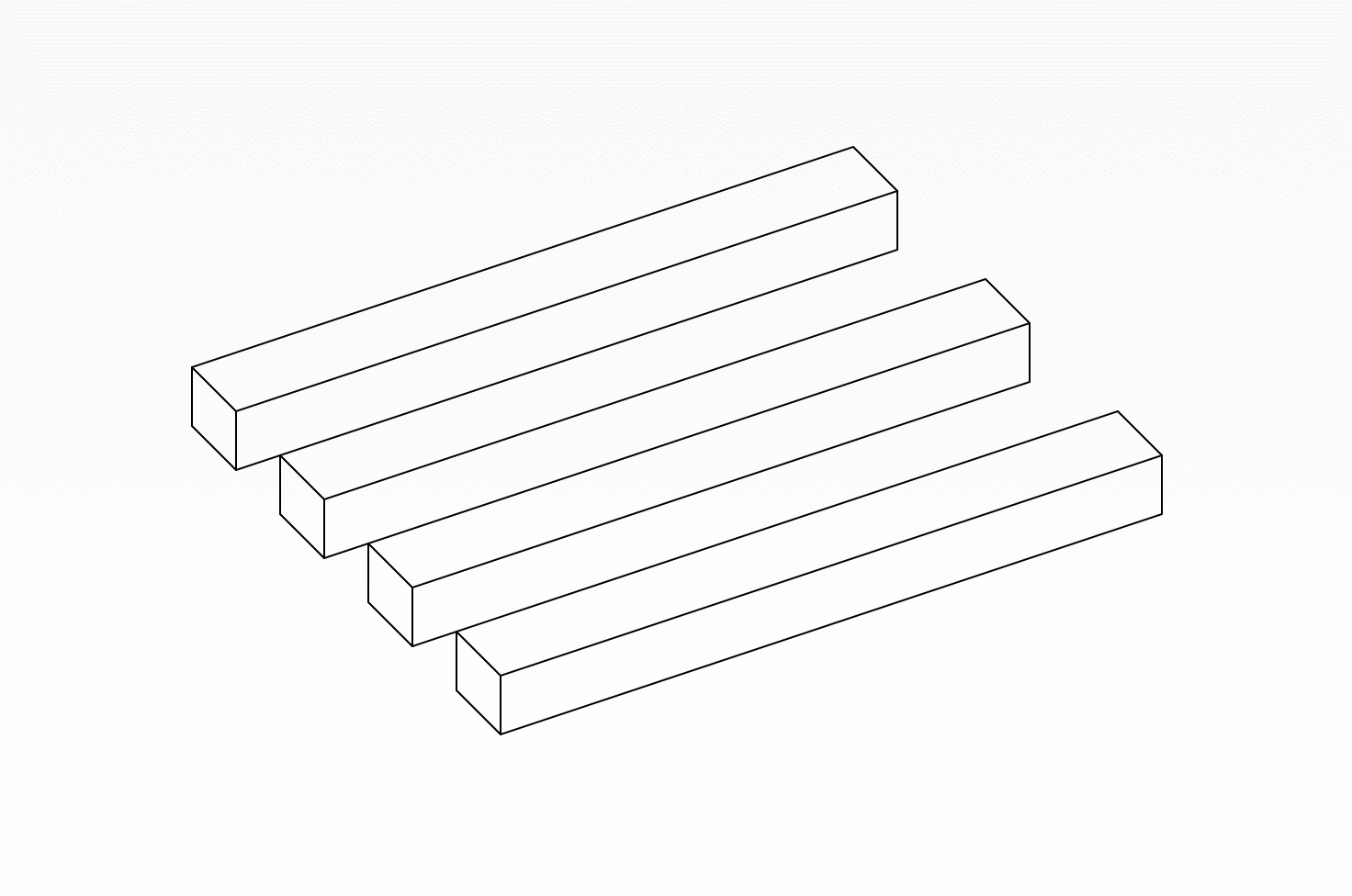
The biggest difference between how biologists and data scientists approach problems is the way they treat data and hypothesis testing.
Hypothesis testing vs. machine learning
Traditionally, scientists look at an outcome (Patient A has cancer but Patient B does not), make a hypothesis (Maybe we can diagnose this cancer with this specific biomarker), and then test that hypothesis using data (Looking for that biomarker in two groups of patients: those who have cancer and those who don’t).
While data scientists using machine learning are guided by this same method, the focus is different. They look at the outcome and make a far looser or broader hypothesis, such as: “Maybe that outcome can be explained by some of the many variables I have in this dataset.” They feed their data into a machine learning algorithm, which might look at billions of potential explanations before automatically honing in on those patterns that best explain the outcome.
This process of using broader hypotheses is foreign to many biologists and often uncomfortable for them. As Alexander says:
“In traditional biology and chemistry, you have a very specific hypothesis, and you lay out a very specific set of experiments to test it. Then once you get there, you have your answer. It's just a couple of simple data points to show that you proved it.
But with data science, you’re often hypothesis-free. You have a loose hypothesis, but then you're doing data-driven analysis to get to an answer.”
Biology’s zealots: Good science or old-fashioned methods?
This is not just a difference in habit. It’s something people have very strong opinions about – beliefs that can border on zealotry. Alexander puts it in religious terms:
“For biologists, it's sacrilegious to not have a hypothesis. That's so contrary to the training and the ethos of being a biologist. It's almost like making people question their scientific religion. Biologists are like, ‘Well, you're a hypothesis-free idiot. Why don't you have a hypothesis?’”
But with new tools and technology that allow us to explore data far more efficiently, machine learning is becoming more accepted. Alexander says:
“There's a lot of evidence of how good machine learning-based analysis is, and how useful it is.”
Machine learning can find far more complex relations in the data
Without algorithms to help them, scientists were limited in the kinds of data analysis they could do. Traditionally, biologists would consider only a very few variables in any given experiment, looking for simple relations in the data, and they would conduct just a handful of experiments.
So it’s no wonder they sometimes find it difficult to understand the potential machine learning offers for their field. Alexander is sympathetic to this view:
“Most bench scientists from the world of biology and chemistry do small numbers of experiments. They do a basic Excel analysis with some simple charts to understand what's going on. Often they think machine learning is just a case of more data points packed into those same bars on the bar chart; they don't quite understand the dimensionality and the complexity that comes with much more data and many more variables.”
Humans often find it difficult to understand high-dimensionality. Geoffrey Hinton explains this succinctly: “To deal with hyper-planes in a 14-dimensional space, visualize a 3-D space and say 'fourteen' to yourself very loudly. Everyone does it.”
But machines are not limited in this way. With increasing computing power and more advanced algorithms, machine learning not only speeds up traditional analysis, but also carries out analyses that would never be possible using only manual methods. Machines can often find correlations between dozens of variables, while human scientists can usually only look at one or two at a time.
Technology allows us to gather more data and move towards broader analysis
Historically, strict hypothesis testing made sense. Gathering data was expensive, so biologists were trained to make sure they only gathered exactly what they needed and to focus on a very specific problem. Alexander acknowledges the reasons behind this narrower perspective:
“Before, biologists had to develop a framework to make experiments work. Without computers. Without high-dimensional data. Without deep learning. You only had enough time and money to collect the very specific things that would prove your hypothesis.
But now it’s so cheap to collect data. While the first whole genome sequence cost $10 billion, the same thing now costs $800.”
The fact that it’s suddenly so cheap and easy to generate data has produced two conflicting schools of thought: some scientists remain more conservative, while others are eager to take full advantage of newer technology. As Alexander explains:
“Basically, the two mindsets are: ‘Why collect all the data you can when you don't know if you need it?’ versus ‘Why not? Because someday you're going to need it.’”
New graduates are leading the perspective shift
Alexander thinks we still need big changes if we’re going to bridge the cultural divide between biology and data science, but he’s optimistic that this shift is already underway. As new graduates enter laboratories, they bring along strong opinions about how to do things more efficiently. As Alexander says:
“Grad students are going to be like, ‘Why are we still doing this by hand? Why don’t we have robots to automate this and data science to analyze it?’”
And it is possible to train people to see each other’s points of view. Alexander went through this shift personally:
“It's all about the framing. In my experience from when I was a biologist, once you train and have experience on the data science side, then you can see it from both angles.”
We need to evolve hypothesis testing, not throw it away
Alexander reminds us that it’s not all about machine learning superseding hypothesis testing. Instead, scientific practices need to evolve and adapt so we can unlock the benefits of tried-and-tested scientific methods combined with the potential of machine learning.
Alexander thinks of this hybrid approach as following a “light” hypothesis: being guided but not limited by your assumptions. As he puts it:
“Microbial sequencing is a good example. Instead of sequencing every microbe I find in a parking lot, I look for microbes in the area I'm interested in. If I want to look for microbes that are good at dealing with gold, then I'd go collect microbes from a gold mine rather than a farmer's field. It's a light hypothesis. You're still not doing things indiscriminately. But you balance it, which is useful.”
It’s not all about talent: The challenges of proprietary formats and legalities
Although it’s still a challenge to find biologists who understand data science – or vice versa – Alexander says the field is more heavily burdened by other factors:
“Very rarely is data science hindered by technical talent.”
Proprietary data formats are the bane of a data scientist’s existence
Data files can be formatted in different ways. Many file types – such as PDF files – have open standards: anyone can implement software to work with PDF files and get access to the details of their internal processes.
By contrast, biotechnology data often uses proprietary or closed data formats, which makes understanding and using this data more of a challenge. Alexander notes:
“We're working very hard to not use anything that stores data in proprietary formats. That's the bane of my data science existence: when there’s some file type I can't open with just any old computer. It drives me nuts. Normalization, standardization, and getting access to that raw data is hard.”
Proprietary software obfuscates internal processing
It’s not only about accessing data; it’s also about understanding exactly what preprocessing steps that data has already been through. Alexander describes the detective work that’s sometimes necessary to discover this:
“It's particularly challenging because oftentimes we don't have access. The software is all proprietary, and we don’t know how the data is processed internally – from raw data to output. Not only do we need to normalize the output of a bunch of different machines, but then we have to figure out what they did to the data before it got to that stage. That's very hard.”
Software vendors use murky legalities to take ownership of data
To help navigate this landscape of proprietary software and data formats, many teams turn to specialist software vendors for help. Alexander has tried this too, but he hasn’t had good experiences. He describes how unwittingly signing a contract can lead to losing ownership of the data itself:
“We've interviewed and assessed a ton of software vendors. We’ve asked how they would provide solutions for this – to make sure we can access the data, and to make sure they don’t end up owning the data just because they stored it in their system. There’s a lot of legal mumbo jumbo around it.”
Are you struggling to find talent or to navigate technology?
We love hearing how different teams are using machine learning in tandem with biotechnology. If you need help or just want to chat, reach out to our CEO directly.